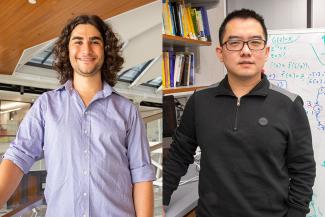
Prof. Jon Tamir and Atlas Wang have received Google Research Scholar Awards for 2023. The Research Scholar Program aims to support early-career professors who are pursuing research in fields relevant to Google.
Jon Tamir received his award for his work on:
Generative Physical Modeling for Blind Imaging Inverse Problems
Description: Deep learning has helped improve the speed and image quality of imaging systems such as MRI scanners. We have previously developed a learning-based framework for robust image reconstruction that combines deep generative neural networks with known imaging physics. However, in many settings the imaging measurement model is not fully correct, for example because of low-fidelity hardware or due to computation constraints. Therefore, in this project we aim to extend our learning framework to the setting where the measurement model is not fully described. We aim to use our approach to handle subject motion and magnetic field inhomogeneity during the MRI scan.
Atlas Wang received his award for his work on:
Efficiently Scale, Adaptively Compute: A Recipe for Training and Using Larger Sparse Models
With increasingly large datasets and models, new computing paradigms must be explored to address two seemingly conflicting goals: keeping scaling our deep networks, while satisfying energy consumption restrictions and demanding constraints for production systems. This proposal aims to accomplish the two goals by efficiently training larger models that can be sparsely and adaptively activated, via the following recipe: (i) Efficiently Scale: we will take a principled approach to warm-initialize a larger model from the pre-trained weights of smaller models, and then continue to train the large sparse model using distributed-friendly, nearly localized training of its different modules; (ii) Adaptively Compute: the trained large model can smoothly navigate a trade-off between its performance and efficiency. When it comes to model reuse for multitasking, the model can also automatically allocate its capacity to heterogeneous tasks. Our technical approaches will synergize and significantly advance state-of-the-art (SOTA) ideas from neural scaling, mixture-of-experts (MoEs), distributed fine-tuning, and adaptive computation. We plan to collaborate with Google teams to benchmark with the SOTA large language models (LLMs) as well as multi-modal pre-trained models. We will also devote efforts to broadening research participation from underrepresented groups.
Jon Tamir is an assistant professor and a Fellow of the Jack Kilby/Texas Instruments Endowed Faculty Fellowship in Computer Engineering the the Chandra Family Department of Electrical and Computer Engineering at The University of Texas at Austin. His research interests include computational magnetic resonance imaging, machine learning for inverse problems, and clinical translation. His PhD work focused on applying fast imaging and efficient reconstruction techniques to MRI, with the goal of enabling real clinical adoption.
Zhangyang “Atlas” Wang is currently the Jack Kilby/Texas Instruments Endowed Assistant Professor in the Chandra Family Department of Electrical and Computer Engineering at The University of Texas at Austin. He is also a faculty member of the UT Computer Science Graduate Studies Committee (GSC), and the Oden Institute CSEM program. Meanwhile, in a part-time role, he serves as the Director of AI Research & Technology for Picsart, developing the next-generation AI-powered tools for visual creative editing. During 2021 - 2022, he held a visiting researcher position at Amazon Search. Prof. Wang has broad research interests spanning from the theory to the application aspects of machine learning (ML). At present, his core research mission is to leverage, understand and expand the role of sparsity, from classical optimization to modern neural networks, whose impacts span over many important topics such as efficient training/inference/transfer (especially, of large foundation models), robustness and trustworthiness, learning to optimize (L2O), generative AI, and graph learning.