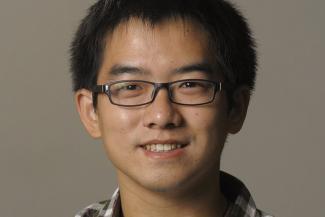
Artificial intelligence and machine learning are among the trendiest technologies in the world right now, and The University of Texas at Austin is rapidly becoming a leader in advancing these concepts as they come to impact more aspects of our daily lives.
A quick primer: Artificial intelligence has been around for a long time, and it is a broad field of computing centered around machines that can perform human-like tasks and solve problems. Machine learning is a branch of artificial intelligence that focuses on programs and devices that can learn and improve on their own, without being explicitly programmed to do so.
In addition to UT Austin’s highly regarded artificial intelligence and machine learning programs, UT’s Machine Learning Laboratory takes a holistic look at the concept, bringing together a community that includes linguists, ethicists, mathematicians, engineers and computer scientists. In 2020, the university was selected to a lead the National Science Foundation’s AI Institute for Foundations of Machine Learning, which also includes the University of Washington, Wichita State University and Microsoft Research.
UT’s AI/ML chops leveled up even further this year when Zhangyang “Atlas” Wang joined the Cockrell School after three years as an assistant professor at Texas A&M University. Wang’s research has garnered recognition from such luminaries in the field as Amazon and IBM.
Wang’s multi-pronged research generally focuses on improving the efficiency of machine learning models. Reducing resources needed for machine learning models makes it easier to export them to mobile devices that lack the processing power currently needed to run these complex technologies.
We sat down with Wang to learn about joining UT Austin in the middle of a pandemic, how it has impacted his research and the most important things he is working on right now:
You arrived at UT last year — what’s it been like to adjust to the job, teaching and working on your research, and doing it all virtually?
It is certainly an unusual and strange time to transition to a new job! I’ve been working at UT for more than half a year, yet I’ve never set foot in my office!
I appreciate that the university, school and department have all been extremely welcoming and helpful. They have very effectively assisted my onboarding process remotely. The campus’ supporting resources are also very useful.
My research is on the computational side, so, fortunately, it is less challenging for me to work remotely with my team, as long as everybody has a laptop. My students and I interact on Slack, Zoom and email on a daily, or even hourly, basis. So far everything seems to be on track, as much as anything can be right now. And I’m tremendously proud of my students who continue to make amazing research progress, even under these challenging circumstances.
Your research focuses on some of the biggest trends in tech: artificial intelligence and machine learning. We’ve heard a lot of hype about these technologies, but it can be hard to see their impact on a day-to-day basis. How is it already impacting our lives, and how will it come do so in the next three to five years?
I would argue that it is easy to see the impact of artificial intelligence on a day-to-day basis. Think about how Google now better understands your text inquiries and can directly search photos with image queries. And look at how much smarter your phone has become over the last five years: face ID or fingerprint access, voice assistant, photo enhancer, etc. All that magic is backed up by the explosive development of AI/ML.
AI/ML have already created greater day-to-day convenience and entertainment. I envision the technologies will make even bigger impacts on our society, economy and environment in the next three to five years. Some of the biggest problems on the agenda that artificial intelligence and machine learning can tackle include but are not limited to: robotics and autonomous driving; smarter home, city and infrastructure technologies; personalized medicine and health care; bioinformatics or drug discovery; and climate change.
All those high-stake domains can benefit from our growingly accurate, robust, efficient, trustworthy and ethically aware AI/ML models. In fact, exciting progress is already being made on them. One great example is Google’s AI offshoot DeepMind’s recent breakthroughs in solving protein structures.
You’re working to improve efficiency in training, or teaching, AI models, which require a tremendous amount of energy. How can we reduce the energy demands of these models?
Training AI models, especially deep networks, includes significant energy consumption, financial costs and environmental impacts. For instance, the carbon footprint of training one deep neural network can be as high as five American cars’ lifetime emissions. More efficient models are also crucial for bringing AI-powered features to more resource-constrained devices, such as mobile phones and wearables.
Our team developed energy-efficient training algorithms for personalizing or adapting deep networks on resource-constrained devices, by combining the best ideas from machine learning, optimization and hardware co-design. For example, when training standard ResNets — which speed up processing by allowing large networks to take shortcuts in order to solve problems — our algorithms reduced energy usage by 80%, without losing much accuracy. Our team recently won second place in the prestigious 2020 IEEE Low Power Computer Vision Challenge, sponsored by top companies such as Facebook, Google and Xilinx.
You recently published a new paper. What were your findings?
New research from our team discovered the existence of highly compact sub-models within the gigantic pre-trained models that demand tremendous resources and power technologies like computer vision and natural language processing. These smaller networks demonstrate the same high efficacy as the original much larger models, when being fine-tuned toward many different tasks.
In other words, we could have trained or tuned such smaller networks from the start and saved a lot of resources. This work, in collaboration with MIT and IBM, reveals a tantalizing possibility in drastically reducing the costs of fine-tuning these pre-trained models that are so prevalent in machine learning right now.
Another major area of emphasis for you is AutoML — or machine learning models that train other machine learning models. This sounds like the beginning of a movie about robots taking over the world. Is that being too paranoid?
Speaking for myself as someone who works in this frontier now, I feel the status quo of AutoML is nowhere near a SkyNet level of scariness. AutoML is still itself a machine learning algorithm that is designed by humans. And it is still in its infancy, with many practical challenges standing in the way of widespread adoption.
But it is exciting to me for many reasons. State-of-the-art AI and ML systems consist of complex pipelines with tons of design choices to make and tune for optimal performance. They also often need to be co-designed with multiple goals and constraints. Optimizing all these variables becomes too complex and high-dimensional to be explored manually. I consider AutoML to be a powerful tool and a central hub in solving those AI/ML design challenges faster and better.
AutoML lets machine learning algorithms try a task millions or billions of times, rapidly going through the trial-and-error process that would take humans much longer to perform. Then it can find an effective route that others can follow to solve similar tasks, without having to repeat the trial-and-error process.
Our team has been contributing to improving the model selection, or neural architecture search (NAS) and algorithm discovery — also known as learning to optimize, or L2O — parts from the full AutoML scope. Asking a machine to take over the trial-and-error process from humans could drastically accelerate our research cycle. This will be especially helpful for organizations that want to use machine learning but don’t know it inside out.